
Oct 19, · This thesis investigates the main issues for the under-performance of CA ASR systems. The work focuses on two directions: first, the impact of limited lexical coverage, and insufficient training data for written CA on language modelling is investigated; second, obtaining better models for the acoustics and pronunciations by learning to transfer between written and spoken blogger.com: Sarah Al-Shareef Title: Arabic Speech Recognition Systems Author: Hamda M. M. Eljagmani Advisor: Veton Këpuska, Ph.D. Arabic automatic speech recognition is one of the difficult topics of current speech recognition research field. Its difficulty lies on rarity of researches related to Arabic speech recognition and the data available to do the experiments Arabic speech recognition has been a fertile area of reasearch over the previous two decades, as attested by the various papers that have been published on this subject. This thesis investigates phoneme and acoustic models based on Deep Neural Networks (DNN) and Deep Echo State Networks for multi-dialect Arabic Speech Recognition
Conversational Arabic Automatic Speech Recognition - White Rose eTheses Online
JavaScript is disabled for your browser. Some features of this site may not work without it. pdf 4. Author Ali, Ahmed Mohamed Abdel Maksoud. Metadata Show full item record. Abstract Dialectal Arabic speech research suffers from the lack of labelled resources and standardised orthography, arabic speech recognition thesis. There are three main challenges in dialectal Arabic speech recognition: i finding labelled dialectal Arabic speech data, ii training robust dialectal speech recognition models from limited labelled data and iii evaluating speech recognition for dialects with no orthographic rules.
This thesis is concerned with the following three contributions: Arabic Dialect Identification: We are mainly dealing arabic speech recognition thesis Arabic speech without prior knowledge of the spoken dialect. Arabic dialects could be sufficiently diverse to the extent that one can argue that they are different languages rather than dialects of the same language. Arabic speech recognition thesis have two contributions: First, we use crowdsourcing to annotate a multi-dialectal speech corpus collected from Al Jazeera TV channel.
We obtained arabic speech recognition thesis level dialect labels for 57 hours of high-quality consisting of four major varieties of dialectal Arabic DAcomprised of Egyptian, Levantine, arabic speech recognition thesis, Gulf or Arabic peninsula, North African or Moroccan from almost 1, arabic speech recognition thesis, hours.
Second, we build an Arabic dialect identification ADI system. We explored two main groups of arabic speech recognition thesis, namely acoustic features and linguistic features. For the linguistic features, we look at a wide range of features, addressing words, characters and phonemes. With respect to acoustic features, we look at raw features such as mel-frequency cepstral coefficients combined with shifted delta cepstra MFCC-SDCbottleneck features and the i-vector as a latent variable.
We studied both generative and discriminative classifiers, in addition to deep learning approaches, namely deep neural network DNN and convolutional neural network CNN. In our work, we propose Arabic as a five class dialect challenge comprising of the previously mentioned four dialects as well as modern standard Arabic. Arabic Speech Recognition: We introduce our effort in building Arabic automatic speech recognition ASR and we create an open research community to advance it.
This section has two main goals: First, creating a framework for Arabic ASR that is publicly available for research. We address our effort in building two multi-genre broadcast MGB challenges. MGB-2 focuses on broadcast news using more than 1, hours of speech and M words of text collected from the broadcast domain.
MGB-3, however, focuses on dialectal multi-genre data with limited non-orthographic speech collected from YouTube, with special attention paid to transfer learning. Second, building a robust Arabic ASR system and reporting a competitive word error rate WER to use it as a potential benchmark to advance the state of the art in Arabic ASR.
Our overall system is a combination of five acoustic models AM : unidirectional long short term memory LSTMbidirectional LSTM BLSTMtime delay neural network TDNNTDNN layers along with LSTM layers TDNN-LSTM and finally TDNN layers followed by BLSTM layers TDNN-BLSTM. The AM is trained using purely sequence trained neural networks lattice-free maximum mutual information LFMMI.
The generated lattices are rescored using a four-gram language model LM and a recurrent neural network with maximum entropy RNNME LM, arabic speech recognition thesis.
Evaluation: The third part of the thesis addresses our effort in evaluating dialectal speech with no orthographic rules.
Our methods learn from multiple transcribers and align the speech hypothesis to overcome the non-orthographic aspects. Our multi-reference WER MR-WER approach is similar to the BLEU score used in machine translation MT. We have also automated this process by learning different spelling variants from Twitter data. We mine automatically from a huge collection of tweets in an unsupervised fashion to build more than 11M n-to-m lexical pairs, and we propose a new evaluation metric: dialectal WER WERd.
Finally, we tried to estimate the word error rate e-WER with no reference transcription using decoding and language features. We show that our word error rate estimation is robust for many scenarios with and without the decoding features. Collections Informatics thesis and dissertation collection. Search ERA.
This Collection. Login Register.
Python in Arabic #39 Speech Recognition التعرف على الكلام -تحويل الكلام الى نص
, time: 11:29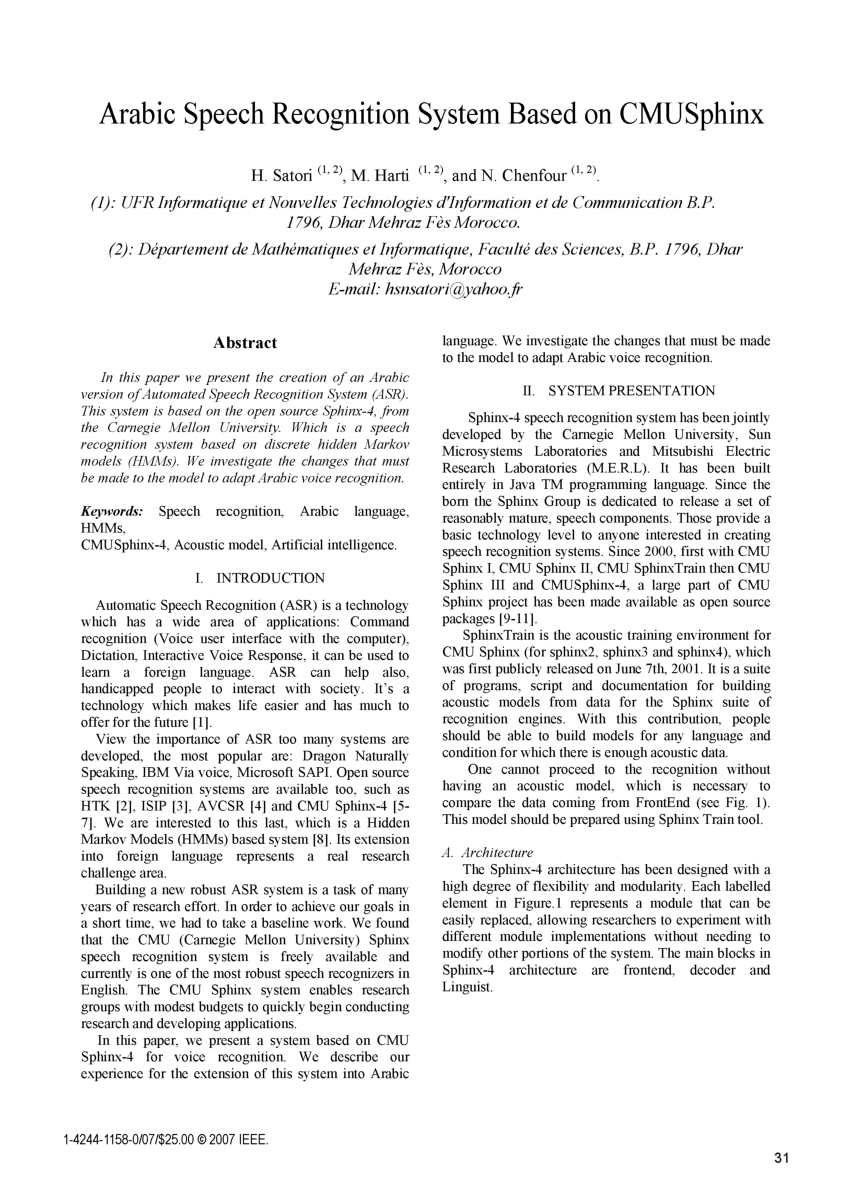
Oct 19, · This thesis investigates the main issues for the under-performance of CA ASR systems. The work focuses on two directions: first, the impact of limited lexical coverage, and insufficient training data for written CA on language modelling is investigated; second, obtaining better models for the acoustics and pronunciations by learning to transfer between written and spoken blogger.com: Sarah Al-Shareef The main theme of this paper is the recognition of isolated Arabic speech phonemes using artificial neural networks, as most of the researches on speech recognition (SR) are based on Hidden Markov This thesis is concerned with the following three contributions: Arabic Dialect Identification: We are mainly dealing with Arabic speech without prior knowledge of the spoken dialect. Arabic dialects could be sufficiently diverse to the extent that one can argue that they are different languages rather than dialects of the same blogger.com by: 8
No comments:
Post a Comment